AI for physics & physics for AI
A central goal of physics is to discover mathematical patterns in data. For example, after four years of analyzing data tables on planetary orbits, Johannes Kepler started a scientific revolution in 1605 by discovering that Mars' orbit was an ellipse. I describe how we can automate such tasks with machine learning and not only discover symbolic formulas accurately matching datasets (so-called symbolic regression), equations of motion and conserved quantities, but also auto-discover which degrees of freedom are most useful for predicting time evolution (for example, optimal generalized coordinates extracted from video data). The methods I present exploit numerous ideas from physics to recursively simplify neural networks, ranging from symmetries to differentiable manifolds, curvature and topological defects, and also take advantage of mathematical insights from knot theory and graph modularity.
Speaker:
Max Tegmark,Institute for Artificial Intelligence & Fundamental Interactions and the Center for Brains, Minds and Machines,MIT;President of the Future of Life Institute
Venue:
Siyuan Hall (W301), Physics Building, PKU
ZOOM: ID: 390597013;Password: 446459
Time:
9:00 am–10:30 am, Oct. 23, 2020 (Friday)
Host:
陈斌 (Bin Chen), bchen01@pku.edu.cn
Biography:
Max Tegmark is a professor doing physics and AI and physics research at MIT as part of the Institute for Artificial Intelligence & Fundamental Interactions and the Center for Brains, Minds and Machines. He advocates for positive use of technology as president of the Future of Life Institute. He is the author of over 250 publications as well as the New York Times bestsellers “Life 3.0: Being Human in the Age of Artificial Intelligence” and "Our Mathematical Universe: My Quest for the Ultimate Nature of Reality". His AI research focuses on intelligible intelligence.
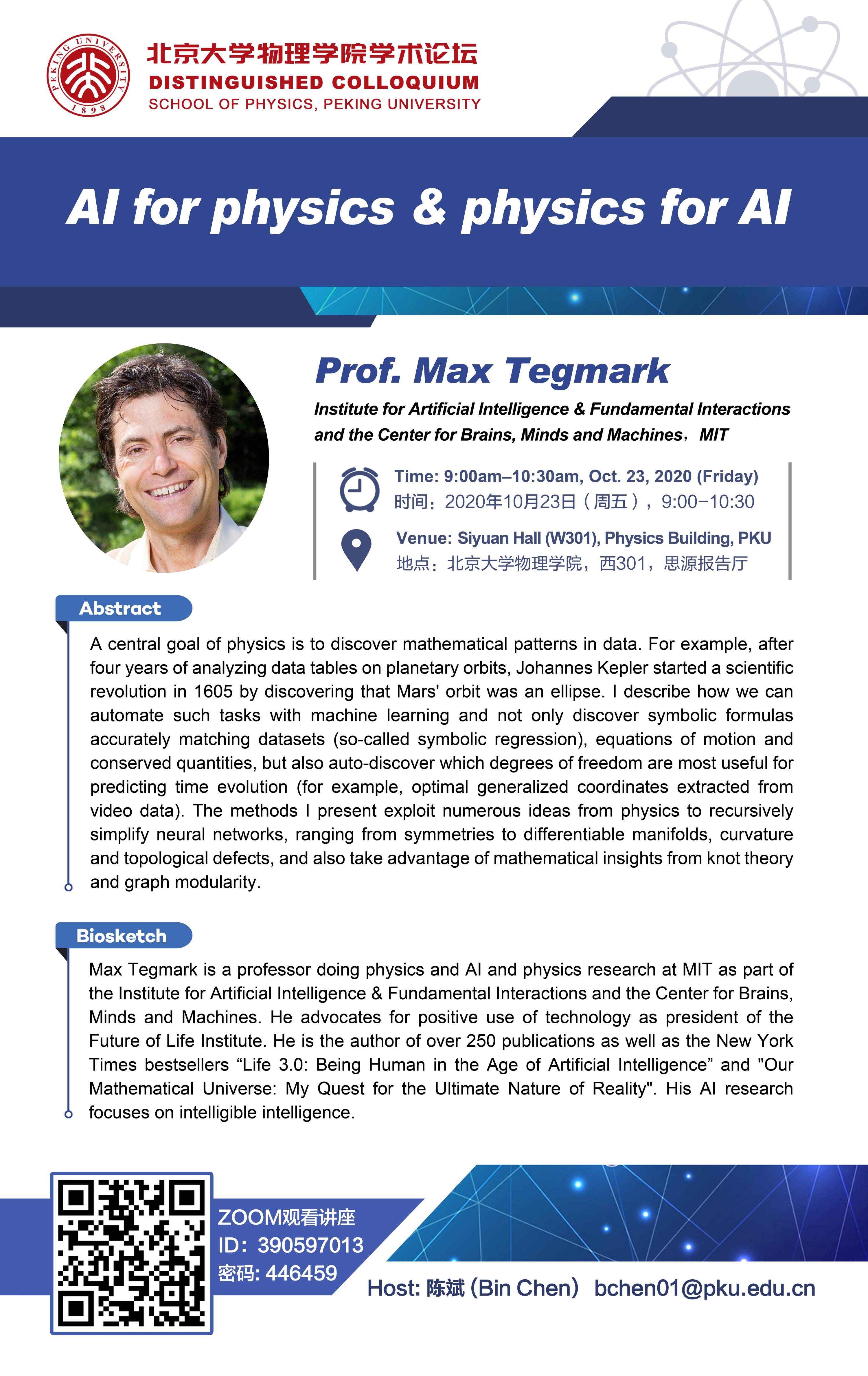